Prof. Shields gives a talk about UQ for ML
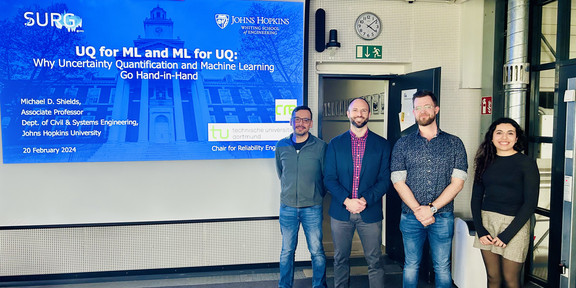
Uncertainty Quantification (UQ) and Machine Learning (ML) play an increasingly important role in physics-based computational modeling. Especially with the recent rise of scientific machine learning (SciML) and physics-informed ML, new computational tools are being harnessed to solve bigger and more challenging problems. Moreover, UQ has become an integral part of any physics-based modeling effort because our models, as carefully developed as they may be, are rife with uncertainties (both epistemic and aleatory) in their parameters, inputs/excitations, and sometimes in the form of the models themselves. When SciML methods are then applied in these applications, additional uncertainties are introduced.
Prof. Shields from John Hopkins Univeristy visited CRE for a week in Feb'24. During his visit, he gave a talk on his work in an event organized by CRE. In this talk, Prof. Shields broadly introduced the interrelated roles that UQ and ML play in physics-based modeling. He specifically distinguished between “UQ for ML” and “ML for UQ” and described the important role that each play in the modern physics-based computational modeling paradigm – demonstrating the role of UQ/ML in various applications of interest, ranging from multi-scale materials modeling to high energy-density physics.
About the speaker
Prof. Michael D. Shields is an Associate Professor in the Dept. of Civil & Systems Engineering at Johns Hopkins University, holds a secondary appointment in the Dept. of Materials Science and Engineering, and is a fellow of the Hopkins Extreme Materials Institute. Prof. Shields conducts methodological research in uncertainty quantification (UQ) and probabilistic modeling for problems in mechanics, materials science, and physics with applications ranging from multi-scale material modeling to assessing the reliability and safety of large-scale structures. He received his Ph.D. in Civil Engineering and Engineering Mechanics from Columbia University in 2010, afer which he was employed as a Research Engineer in applied computational mechanics at Weidlinger Associates, Inc. He joined the faculty at Johns Hopkins in 2013. For his work in UQ, Prof. Shields has been awarded the ONR Young Investigator Award, the NSF CAREER Award, the DOE Early Career Award, and the Johns Hopkins University Catalyst Award. Prof. Shields and his group also develop the open-source UQpy (Uncertainty Quantification with Python) sofware, which is a general toolbox and development environment for UQ in computational, mathematical, and physical systems.